In the vast landscape of data analytics, there exists a critical yet often overlooked stage: data preparation and manipulation. Picture this: you’ve got a mountain of raw data—imagine it as a tangled web of wires, each carrying valuable information but wrapped in knots of confusion. This raw data, unrefined and chaotic, holds the potential to unlock insights that can drive business decisions and innovation. But before we can harness its power, we must first embark on the journey of transforming it from chaos to clarity.
Raw data, much like a messy room, requires tidying up before it can be of any use. This process involves cleaning, preprocessing, and manipulating the data into a format that’s not only usable but also reliable. Think of it as organising a cluttered desk—sorting through papers, discarding irrelevant ones, and arranging the rest in a logical manner.
Data cleaning is akin to dusting off the cobwebs, removing inconsistencies, and fixing errors that may have crept into the dataset. It’s about ensuring that the data is accurate and free from any imperfections that could skew our analysis—just like polishing a gemstone to reveal its true brilliance.
Once the data is clean, we move on to preprocessing, where we prepare it for analysis by standardising formats, handling missing values, and scaling variables. This step is like preparing ingredients before cooking a meal—chopping vegetables, marinating meat, and measuring out spices to ensure everything is ready for the recipe.
But our work doesn’t stop there. Data manipulation involves transforming the data to extract meaningful insights. This could include aggregating, merging, or reshaping the data to uncover patterns and relationships—much like a sculptor molding clay into a work of art, shaping it until the desired form emerges.
Ultimately, the goal of data preparation and manipulation is to transform raw data into a refined and structured format that’s ready for analysis. It’s about turning chaos into clarity, unlocking the hidden potential within the data to drive informed decision-making and innovation.
Now that we’ve laid the groundwork, it’s time to delve deeper into the world of data preparation and manipulation.
Read on to explore the top 10 mistakes people make in this crucial stage of the data analytics process——> link to website blog…and closing paragraph for the newsletter.
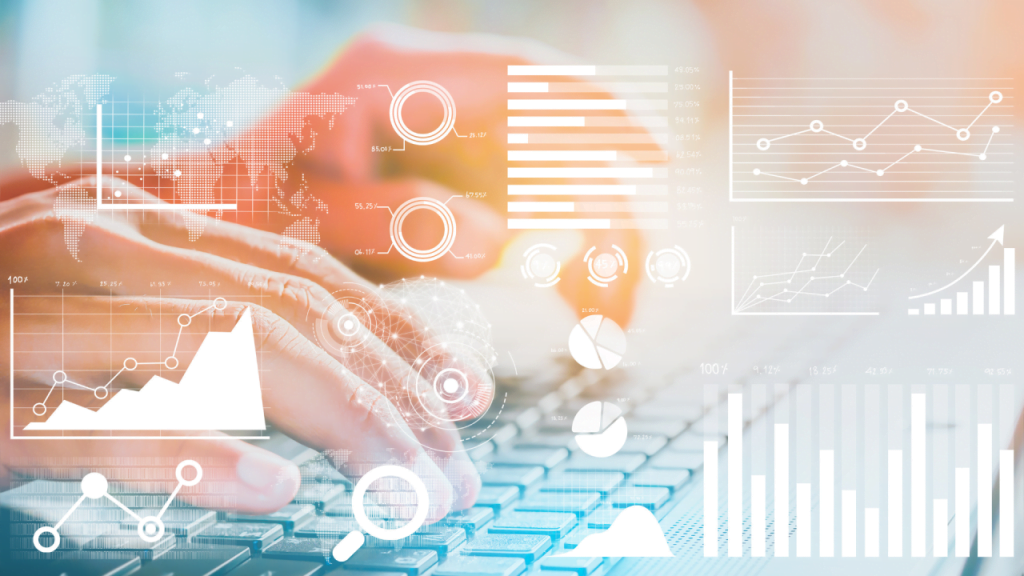
I know, you’re here for the fun part – the analysis, the data visualisation, the insights, the power and the glory….but if you don’t do the grind, you wont do the find…
Here are the top 10 mistakes people make in relation to “Data Preparation and Manipulation”:
1. Skipping Data Cleaning
Analysis based on uncleaned data can lead to inaccurate insights and flawed decision-making. It’s like trying to bake a cake without sifting the flour—expect a lumpy outcome and disappointed data consumers.
2. Overlooking Data Validation
Failure to validate data integrity can result in erroneous conclusions and wasted resources. It’s a bit like driving to a new destination without setting your sat nav first – prepare for a long journey to nowhere, blissfully unaware you are lost.
3. Not Addressing Missing Values
Ignoring missing data can skew analysis results and compromise the reliability of findings. It’s like starting a puzzle with missing pieces—your final picture will haves gaps and your data story will be incomplete. You and your audience will find it puzzling to understand..
4. Using Manual Processes Instead of Automation
Manual data manipulation increases the risk of errors, consumes time, and hampers scalability. Tools like Python pandas, R tidyverse and Tableau Data Prep Flows can streamline tasks and enhance efficiency. It’s a bit like chopping wood with a butter knife— possible but tedious, inefficient, and likely to result in a broken tool and a broken soul…and a lot of wasted time.
5. Applying Incorrect Transformation Techniques
Incorrect transformations can distort data relationships and lead to misleading interpretations. Understanding when to use techniques like normalisation, standardisation, or logarithmic transformation is crucial. Ever tried to fit a square peg into a round hole? No matter how hard you push, it just won’t fit, and you’ll end up with a mismatched mess. The problem with data transformation is that it will work, and you can make it fit, even if it just isn’t the right tool for the job.
6. Ignoring Data Format Compatibility
Incompatible data formats can disrupt integration efforts and impede analysis. Tools like Apache Spark, Talend, Apache Hop and Tableau Data Prep can help convert data into compatible formats. It’s like speaking English to someone who only understands French —communication breaks down, nothing gets accomplished, barriers grow, channels expand, free trade agreements get abolished, I could go on.
7. Failing to Document Data Transformation Steps
Lack of documentation makes it difficult to replicate analysis and verify results, reducing the credibility of findings. It’s like cooking a culinary masterpiece without writing down the recipe— you wont remember all the ingredients when asked (a serious food crime that I was often guilty of as a student) and you’ll struggle to recreate it in the future (which some of my friends felt wasn’t a bad thing as a student, but not so good as a data analyst driving decisions for senior management…).
8. Not Considering Data Scalability
Data manipulation techniques that work for small datasets may not scale effectively, leading to performance issues and resource constraints. It’s like patching up an old pair of jeans instead of getting a new pair when you realise that actually, they don’t fit anymore anyway.
9. Underestimating the Importance of Data Governance
Poor data governance practices can result in data inconsistencies, compliance violations, increased organisational risk, time wasted, angry users, violence and many late nights wondering where it all went wrong whilst backtracking and unpicking locks with broken keys jammed inside.
10. Overcomplicating Data Preparation
Overly complex data preparation workflows can hinder understanding and maintenance, making it challenging to adapt to changing requirements and scale efficiently. Simplifying processes and focusing on essential transformations can enhance effectiveness and maintainability. You wouldn’t build a Swiss watch every time you wanted to tell the time…
In conclusion people, let’s not be daft about it. Data prep and manipulation isn’t a walk in the park, but it’s also not brain surgery.
Remember, just like trying to make a cuppa without boiling water, skipping crucial steps or using the wrong tools will land you in a right pickle.
So, keep it simple, stay sharp, and remember, if all else fails, there’s always a backup plan: blame it on the intern!